
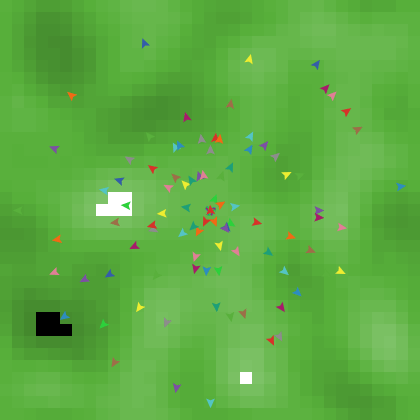
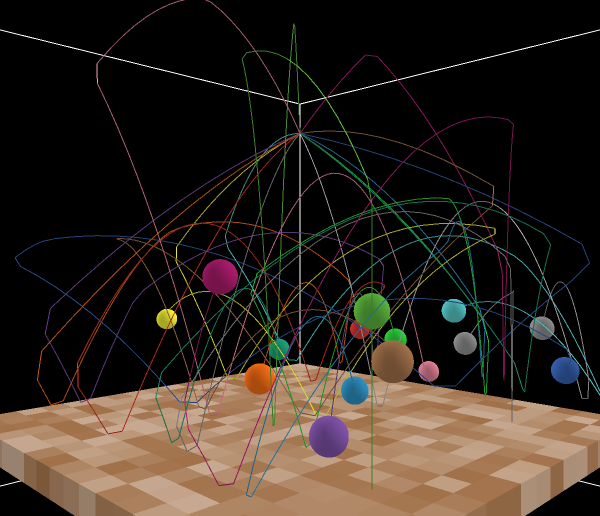
The agent represents its current situation in terms of perceived affordances that develop through the agent’s experience. Furthermore, I propose a Bottom-up hiErarchical sequential Learning model based on the CCA, which is also called BEL-CA, as a solution for an autonomous agent learning hierarchical sequences of behaviors and acquiring capabilities of self-adaptation and flexibility. Following these drives, the agent autonomously learns regularities afforded by the environment, and constructs causal perception of phenomena whose hypothetical presence in the environment explains these regularities. In addition, I present two forms of self-motivation: successfully enacting sequences of interactions (or called autotelic motivation), and preferably enacting interactions that have predefined positive values (or called interactional motivation). Instead, I propose a way for the agent to autonomously encode the interaction experiences and reuse behavioral patterns based on the agent’s self-motivation implemented as inborn proclivities that drive the agent in a proactive way. Accordingly, I am not proposing an algorithm that optimizes exploration of a predefined problem-space to reach predefined goal states. In contrast with traditional cognitive architectures, the introduced model neither initially endows the agent with prior knowledge of its environment, nor supplies it with knowledge during its learning process. Meanwhile, the CCA allows a self-motivated agent to autonomously construct the perception of the environment and acquire capabilities of self-adaption and flexibility to generate proper behaviors to tackle with diverse situations in interacting with the environment. In this dissertation, I propose a computational model of Constructivist Cognitive Architecture (CCA) as a way towards simulating the early learning mechanism of infants’ cognitive development based on theories of enactive cognition, intrinsic motivation, and constructivist epistemology. Seeking ways to explain the learning mechanism behind infants’ early cognitive development and try to replicate some of these abilities that babies have for an autonomous agent have become a focal point of recent efforts in robotics and AI research. In most traditional Artificial Intelligence (AI) approaches, learning is usually insufficient, with various biases, and lacks of flexibility. For most artificial agents (and robots), acquiring such abilities is overwhelming. These abilities of sense-making and knowledge construction of the environment set them apart from even the most advanced autonomous robots. Especially in the initial phase of cognitive development, they exhibit amazing abilities to generate novel behaviors in unfamiliar situations, and explore actively to learn the best while lacking extrinsic rewards from the environment. Infants are excellent at interacting with the environment.
